Are you curious about how Spotify always seems to know exactly what song you’re in the mood for? It’s all thanks to Spotify’s advanced recommendation system powered by AI algorithms. This innovative technology is what makes Spotify stand out in the world of music streaming.
So, how does Spotify’s algorithm work? Let me break it down for you. The algorithm analyzes a wealth of data, including your listening history, preferences, and behaviors, to create a personalized music experience just for you. It’s like having a virtual DJ that knows your music taste inside out.
Spotify’s recommendation system is designed to maximize user engagement. By providing tailored music suggestions that align with your interests and preferences, Spotify aims to keep you hooked to the platform and enhance your overall music discovery experience.
To gain a better understanding of Spotify’s algorithm and how it revolutionized the way we discover music, let’s dive into its impact on the music industry and the technology behind its personalized playlists.
The Evolution of Music Discovery: Spotify’s Impact
Spotify has undoubtedly revolutionized the way we discover music in the digital age. Through the introduction of advanced data analysis and personalized recommendations, the platform has transformed the music streaming landscape. Unlike previous platforms that offered generic playlists, Spotify utilizes a sophisticated music streaming algorithm to curate personalized playlists tailored to individual preferences.
By analyzing patterns in user behavior, Spotify’s algorithm creates a musical ecosystem where tracks and users are intricately interconnected. This interconnectedness not only enhances the personalized music discovery experience but also allows users to explore new genres, artists, and tracks that align with their individual taste.
Spotify’s personalized playlists are the epitome of the platform’s commitment to enhancing user engagement and satisfaction. These playlists go beyond mere song recommendations, taking into account factors such as mood, genre, and even the time of day to curate a seamless and immersive listening experience for each user.
Understanding Spotify’s Data Layers and Shared Models
Spotify’s recommendation system is powered by a comprehensive data analysis framework that delves into user preferences, behaviors, listening history, and interactions with the platform. This extensive dataset forms the foundation for the recommendation models and personalized playlists that enhance the user experience.
One of the techniques employed by Spotify is collaborative filtering, which involves connecting users and tracks based on shared preferences. Collaborative filtering allows the algorithm to identify frequently playlisted songs, which form clusters based on their co-occurrence. These shared models capture the collaborative approach to the recommendation, resulting in more accurate and personalized music suggestions.
By leveraging vast amounts of user data, Spotify’s recommendation system continuously learns and adapts to individual tastes and preferences. The data layers and shared models are crucial components that contribute to the effectiveness and reliability of Spotify’s music recommendation technology.
The Integration of Data Layers in Spotify’s Recommendation System
In Spotify’s recommendation system, the integration of various data layers plays a crucial role in providing personalized music recommendations to users. By leveraging extensive data analysis, Spotify’s algorithm generates accurate insights into users’ music preferences and behaviors.
The data layers encompass a wide range of sources, including user behaviors, preferences, playlist creation, and track listening history. By analyzing this data, Spotify’s algorithm gains valuable insights into individual tastes and creates tailored music experiences for each user.
This integration allows the recommendation algorithm to curate a refined selection of songs that align with users’ personal preferences. Spotify’s ability to connect with users on a deeper level through accurate data analysis sets it apart from traditional music streaming platforms.
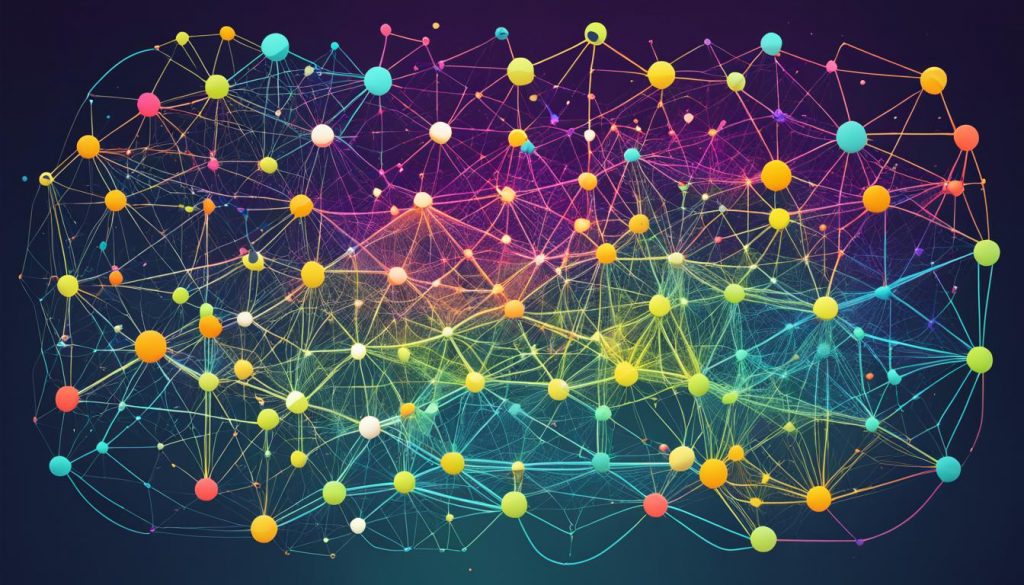
The Role of Content-Based Filtering in Spotify’s Algorithm
One of the key components of Spotify’s algorithm is content-based filtering. This technique allows the algorithm to understand the sonic characteristics of different tracks, enabling it to provide more nuanced recommendations based on the content of the songs.
Spotify’s algorithm utilizes a combination of metadata analysis and audio analysis to gain insights into each song. Artist-sourced metadata, including track title, release title, artist name, genre, and more, is analyzed to understand the context and attributes of the tracks.
The algorithm also performs raw audio analysis to extract objective and perceptual features of the track. This includes analyzing factors such as danceability, energy, valence, and more. By considering both metadata and audio analysis, Spotify’s algorithm can generate more accurate recommendations that align with the preferences and interests of each user.
This content-based filtering approach allows Spotify to provide users with a diverse range of song recommendations, taking into account various song characteristics and attributes. By understanding the content of the songs, Spotify can create a more personalized and engaging music streaming experience for its users.
Exploring Collaborative Filtering in Spotify’s Recommendation System
Collaborative filtering is a crucial component of Spotify’s recommendation system, contributing to its highly personalized music discovery experience. Through collaborative filtering, Spotify connects users and tracks based on shared preferences and behaviors, creating clusters of songs that are frequently playlisted together. These clusters represent shared musical preferences among users, allowing Spotify to recommend tracks based on similar user behaviors.
The proximity of tracks on the music map reflects their similarity, meaning that users who enjoy one song are more likely to appreciate neighboring songs. This collaborative approach enhances the personalization of Spotify’s recommendations, making it more likely for users to discover new songs and artists that align with their tastes.
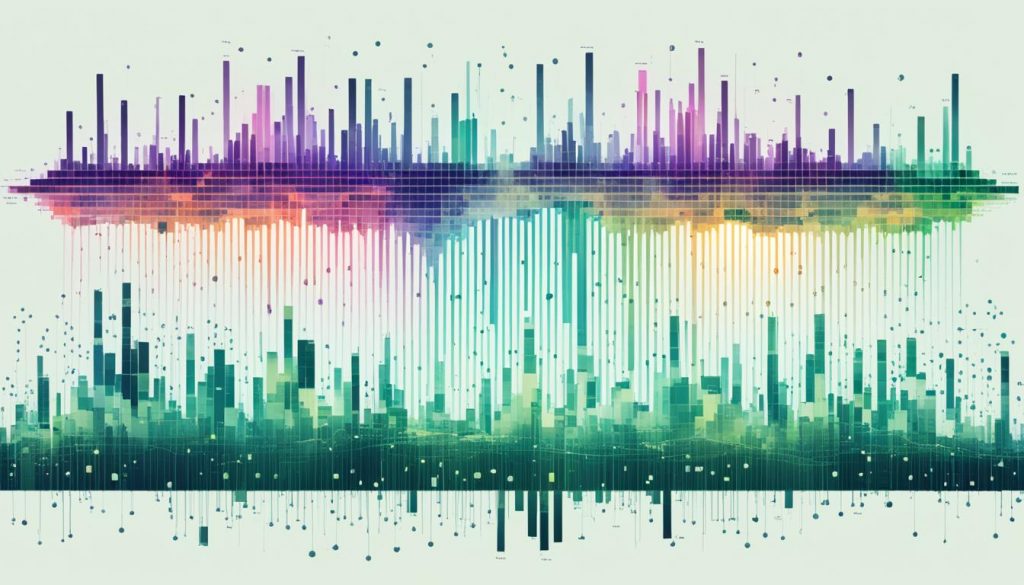
By leveraging collaborative filtering, Spotify achieves a deeper understanding of user preferences and creates more accurate and targeted music recommendations. This approach enables users to explore a wide range of musical genres and styles, gaining exposure to songs that they might not have discovered otherwise. Collaborative filtering plays a vital role in enhancing the overall music discovery experience on Spotify, fostering a sense of connection between users and the vast library of tracks available on the platform.
The Power of Spotify’s Recommendation Algorithm
When it comes to music streaming, Spotify’s recommendation algorithm is a game-changer. This powerful algorithm connects users with the music they love by providing personalized recommendations. By analyzing extensive user data, Spotify’s algorithm creates a unique and enjoyable music streaming experience for each individual.
The goal of Spotify’s algorithm is to maximize user engagement. The algorithm takes into account various factors such as listening history, preferences, and behaviors to generate recommendations that are tailored to each user’s taste. These personalized recommendations not only keep users engaged but also foster a sense of connection and loyalty to the platform.
A Data-Driven Approach
To create accurate and personalized recommendations, Spotify’s algorithm relies on a solid foundation of user data. The algorithm collects and analyzes data on user behaviors, preferences, playlist creation, and track listening history. By integrating this wealth of data, Spotify’s algorithm is able to curate a refined and tailored music experience for each individual user.
This data-driven approach enables Spotify’s recommendation algorithm to continuously learn and adapt to the evolving preferences of users. Machine learning models are employed to optimize user retention and generate revenue, ensuring that the recommendations align with both user preferences and the platform’s goals.
Recommendations are considered “good” when users engage with the suggested songs. This engagement is measured through metrics such as longer listening sessions and increased interactions with the platform. By delivering recommendations that resonate with users, Spotify’s algorithm encourages them to spend more time listening to music and exploring the platform.
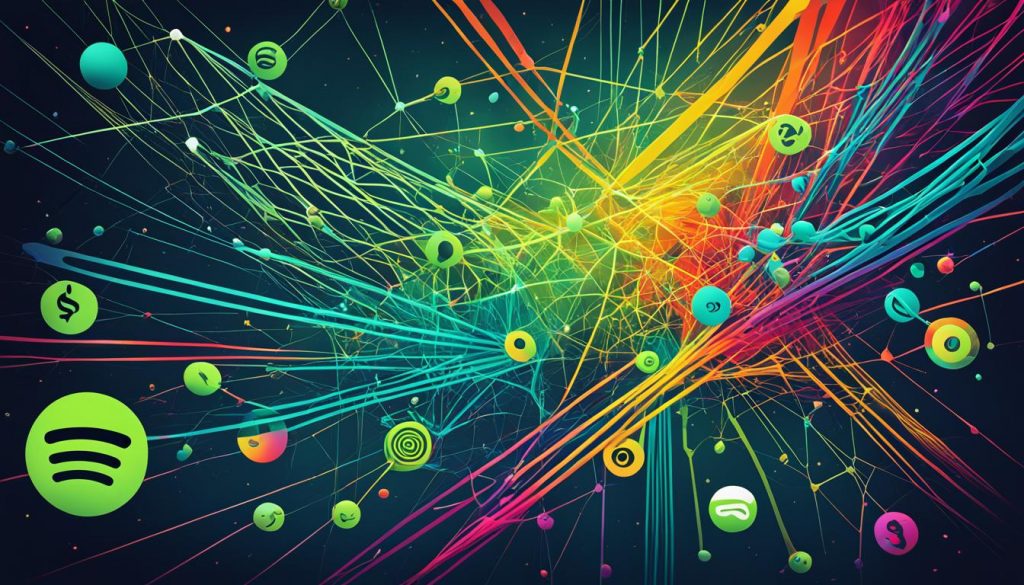
Strategies for Musical Artists in the Age of Spotify
The rise of streaming platforms like Spotify has revolutionized the music industry, providing both challenges and opportunities for artists. In this section, we will explore effective strategies that artists can employ to navigate the digital landscape and maximize their exposure, revenue, and success.
Focusing on Singles
When it comes to releasing music on Spotify, artists can optimize their strategy by focusing on singles rather than full albums. The algorithm tends to promote individual songs, making it more likely for them to be discovered by listeners. By strategically releasing singles and promoting them through social media and online platforms, artists can increase their chances of gaining traction and attracting a wider audience.
Targeting a Niche Audience
One effective strategy for artists is to identify and target a niche audience. By tailoring their music to the preferences and interests of a specific group of listeners, artists can establish a dedicated fanbase. This targeted approach allows artists to build a strong connection with their audience, leading to increased engagement, loyalty, and support.
Choosing the Right Distribution Method
Artists have the option to distribute their music independently or sign with a larger distributor. Each approach has its advantages and considerations. Independent distribution provides artists with more ownership and control over their music, while signing with a larger distributor offers structured publicity and access to a wider network. Artists should carefully evaluate their goals, resources, and priorities to make an informed decision that aligns with their career trajectory.
Diversifying Revenue Sources
While streaming revenue from platforms like Spotify plays a significant role, artists should also explore other revenue sources to sustain their careers and increase their income. Live performances and virtual concerts have become increasingly popular and can provide a substantial source of revenue. Additionally, artists can monetize their brand through merchandise, sponsorships, and partnerships. Diversifying income streams mitigates the reliance on streaming revenue and provides artists with more financial stability.
To summarize, a successful music career in the age of Spotify requires artists to adapt and employ effective strategies. By focusing on singles, targeting a niche audience, choosing the right distribution method, and diversifying revenue sources, artists can optimize their visibility, engagement, and streaming revenue. Embracing the opportunities presented by streaming platforms while strategically navigating the digital landscape is key to long-term success in the music industry.
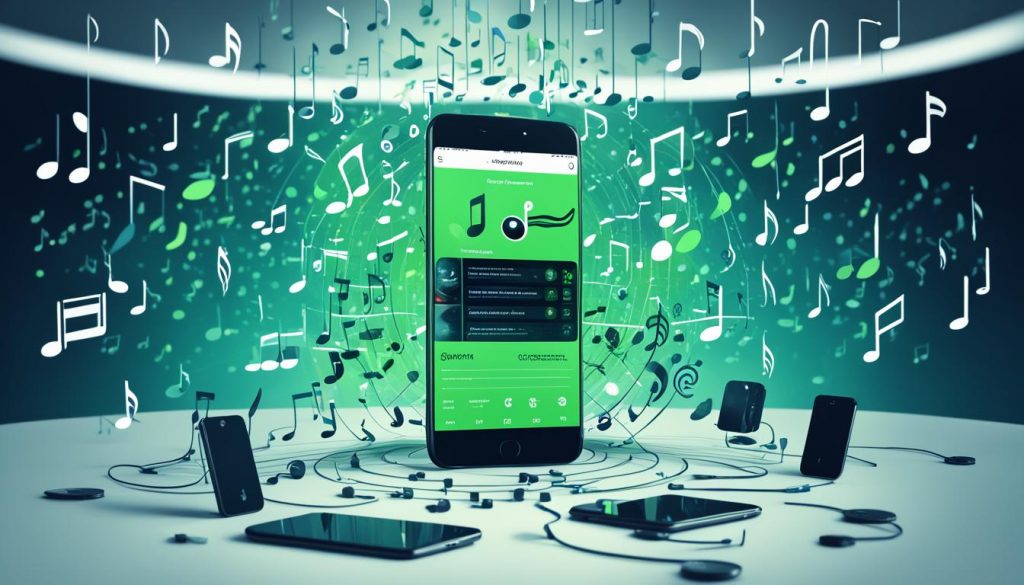
The Future of Music Distribution in the Algorithmic Age
As algorithmic recommendation systems continue to shape the music industry, there are ongoing discussions about their impact on artists and the future of music distribution. Personalized algorithms, like those used by Spotify, have revolutionized the way listeners discover music and connect with artists. These algorithms analyze user data to curate tailored playlists and recommendations, increasing the visibility of artists and expanding their fan base.
However, it is important to note that while these algorithms can enhance artists’ exposure, they do not guarantee widespread fame or significant financial success. Artists must recognize that the dynamic landscape of streaming platforms calls for continuous adaptation and innovation. In addition to leveraging algorithms, artists can explore new opportunities, such as virtual concerts and alternative revenue streams, to diversify their income sources and connect with their audience in unique ways.
While the music industry embraces the benefits of algorithmic recommendations, it is crucial to strike a balance between personalized algorithms and the creative endeavors of artists. By finding this balance, artists can navigate the ever-changing landscape of music distribution, leveraging technology to amplify their artistry and connect with listeners on a deeper level. As the industry evolves, it is essential to prioritize both the personalization offered by algorithms and the human touch that artists bring to their craft.